Predicting nodal status in male breast cancer using mammogram based radiomic metrics
Introduction
Male breast cancer (MBC) is a rare disease, comprising less than 1% of all breast cancer cases in the USA and less than 0.1% of cancer related deaths in men (1). While the incidence of female breast cancer (FBC) has been decreasing since the year 2000, the juxtaposed incidence of MBC has risen by 26% over the last 25 years. Dedicated breast cancer research in males would be valuable (2). Some of the hurdles include the lack of sizable patient cohort from prospective studies owing to the rarity of MBC and the frequent exclusion of male patients from breast cancer clinical trials (1). Therefore, guidelines in the treatment of MBC are extrapolated from the data generated from FBC trials or based on small retrospective cohorts (2). Further, data derived from retrospective studies are observational and hence subjective (2). Nevertheless, nodal status appears to remain a key prognostic discriminant and is important for tailoring treatment in MBC (3).
Currently, imaging is used as a non-invasive method in a preoperative setting in the evaluation of MBC. Diagnostic mammography remains the mainstay of imaging but ultrasonography (US), computed tomography (CT), magnetic resonance imaging (MRI) and positron emission tomography (PET) can play a key role in diagnosis and staging.
Despite recent advances in imaging there remains a low diagnostic sensitivity for axillary lymph node (ALN) involvement resulting in understaging of the disease even when lymph nodes are visible on imaging (Figure 1) (4). A systematic review estimated the average sensitivity for positivity of US in patients with nonpalpable axillary nodes to be 44–61% and specificity for positivity at 75–86% in all patients (5). Currently, if clinical and US evaluation suggest nodal metastases on the basis of size or abnormal morphology, US-guided biopsy [fine-needle aspiration cytology (FNAC) or core biopsy] of abnormal nodes is undertaken, which detects 45% of metastases (5). However, there are a significant number of false negatives.
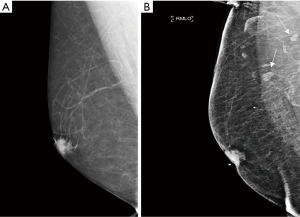
The current study evaluates only the primary tumor to identify radiomic metrics capturing a variety of tumor phenotypes such as size, shape and radiological texture from mammograms which could predict the presence of nodal metastases. We report a reasonable performance [area under the curve (AUC) =0.71] in discriminating pathologically node negative vs. node positive male patients. Currently, we are limited by no availability of nodal imaging in a large proportion of our retrospective cohort. However, we hope to address this in future and prospective studies. Therefore, any method that improves upon a more accurate detection of nodal disease would be important. Accurately assessing pathological nodal status prior to surgery can help clinicians optimize treatment strategies by identifying patients who may be eligible for neoadjuvant chemotherapy/endocrine therapy or even omission of sentinel node biopsy and enrollment in clinical trials.
Radiomics is an emerging field of quantitative medical imaging that involves converting medical images into mineable and scalable high-dimensional data sets derived primarily from standard of care imaging. Predictive models based on the extracted datasets along with additional clinical, pathologic and laboratory data have been shown to improve upon the accuracy of cancer diagnosis, prognosis and prediction (6,7). The radiomic process involves lesion segmentation from clinically obtained imaging, feature extraction, and finally model building. The radiomic features that are extracted reflect the heterogeneity of the tumor by calculating pixel intensities and their spatial distribution over a region of interest (ROI). Both quantitative and mathematical descriptions of the spatial distribution of signal intensities are referred to as texture analysis.
Numerous radiomic studies in FBC using mammography and breast MRI have been published (8-11). Several studies have established MRI imaging features combined with radiomics can be used to predict molecular subtypes, luminal A (P=0.004), HER2/neu enriched (P=0.00277) and basal like (P=0.0117) (11) and predict imaging biomarkers predicting pathways associated with survival (6). Textural features obtained from mammograms have also been used to predict BRCA1/2 probability from textural parenchymal differences not detectable by standard radiologic interpretation (9).
There are several studies evaluating mammography, MRI and US to evaluate axillary node status in FBC. For example, Tan et al. (12) developed a mammogram-based radiomics nomogram by integrating radiomic metrics with clinical risk factors to predict preoperative axillary metastasis and reported an AUC 0.876 and 0.862 in the primary and validation cohorts. Dong et al. (13) showed that using radiomics in DWI and joint fat sat T2 MRI can preoperatively predict sentinel lymph node (SLN) metastasis in FBC with an AUC of 0.80 in the validation set. Using deep learning in combination with radiomic metrics from the US of 1,280 patients breast cancer patients Guo et al. (14) identified patients with positive sentinel node metastasis with a sensitivity of 98.4% and those without metastasis with a 97% negative predictive value. To our knowledge, there have been no studies evaluating omitting sentinel node biopsy based on radiomic nodal assessments.
Radiomic features have been used to classify mammographic breast parenchymal complexity. These radiomic features in combination with breast density and body mass index (BMI) have been shown to help stratify breast cancer risk assessment (15).
To our knowledge, there has been no published radiomic analysis in male patients with breast cancer. Therefore, the aim of this study was to develop a mammography-based radiomics platform by performing a retrospective evaluation of the radiomics (tumor texture, size and shape metrics) of primary lesions in subjects MBC. Specifically, we wanted to explore the feasibility of using radiomic signatures from the primary lesion to discriminate pathologically node positive vs. node negative MBC patients based upon diagnostic mammograms.
We present the following article in accordance with the Materials Design Analysis Reporting (MDAR) reporting checklist (available at http://dx.doi.org/10.21037/abs-20-103).
Methods
Patient data
The retrospective study was conducted in accordance with the Declaration of Helsinki (as revised in 2013). The study was approved by the Western Institutional Review Board to exempt the ethics review with the exemption number (B4-Exemption-Moorthy). Informed consent was also waived. A total of 75 MBC patients, from our breast cancer database, who underwent surgical treatment at a single institution (Arizona Center for Cancer Care) between 2009–2020 were included in this study. The inclusion criteria included men diagnosed with a pathologically confirmed breast cancer with access to their 2D digital mammograms prior to their surgical intervention with complete pre surgical and surgical information. Patients in whom the mammograms were not available (n=15) were excluded. All digital mammography images were obtained from the picture archiving and communication systems (PACS). Clinicopathologic and immunohistochemical factors from core biopsy were acquired from the prospectively maintained center database. Demographic variables included age, sex, and ethnicity. Relevant prior clinical history of cancer was also recorded. Clinical and pathological factors such as clinical T stage, clinical nodal status, estrogen receptor (ER), progesterone receptor (PR), Ki67, human epidermal growth factor receptor (HER/2-neu), surgical management, adjuvant therapy, local regional recurrence rate, disease free survival and overall survival data were collected.
Mammography acquisition
All patients included in the analysis had 2D digital mammograms [craniocaudal (CC) and mediolateral oblique (MLO)]. All digital mammograms were acquired per the site’s standard of care procedures for exposure settings and the images were stored in PACS in DICOM format. De-identified images were curated and archived onto an open source image segmentation software for segmentation and feature extraction as described below.
Mammographic inspection and tumor segmentation of tumor lesions
A single breast specialist (BM) with over 15 years of experience in mammography reviewed all images. The reader was NOT blinded to the side or the location of the tumor. The mammographic images (CC and MLO views) from all 60 subjects (10 were clinically node positive, while 50 were clinically node negative) were available for evaluation While a subset of patients (n=7) had a 3D mammogram, a 2D radiomic analysis was conducted to maximize the cohort sample size. Consequently, the ROI was marked on the 2D component of the mammogram or reconstructed 2D mammogram. All tumors were detectable on 2D mammograms. This cohort was acquired retrospectively before 3D mammo was widely available. Cancer Imaging Phenomics Toolkit (CaPTk) can be used to mark ROI on 3D images. A 3D dataset was not available for all the cases included in this cohort. Though 3D tomosynthesis is the primary modality being used for screening in many centers, there are logistical issues that arise when using it in radiomics. As this was a multicenter study, there was no consistent imaging protocol that was adopted. While 3D evaluation may improve the accuracy of radiomic evaluation, in some instances 2D evaluation may produce similar accuracy (16-18).
All had the presence of the mass lesions (primary tumor) on mammography. Any features such as suspicious calcifications, skin thickening, or nipple retraction were not captured unless there was an overlap with the segmented primary tumor. Any mammographically suspicious lymph nodes were not included in the imaging. For each patient, a single lesion was identified on both the CC and MLO view mammograms for radiomic analysis.
The tumor lesions on the mammograms were segmented using a 2D ROI that was placed around the whole lesion in both the CC MLO view using ITK-SNAP (open source software; http://www.itk.snap.org) for image segmentation (19,20). The resultant ROIs from the ITK-SNAP segmentations were saved for transfer, processing and radiomic analysis (Figure 2). According to Huang’s and Dong’s study (13,21), a radiomics signature was concluded by radiomics features that extracted from primary tumor, which was used to predict lymph nodes metastasis and show a significant performance. Therefore, it is reasonable that we choose the primary tumor as the segmentation region. In future and prospective studies when we have access to dedicated nodal imaging, we will address this specific issue.
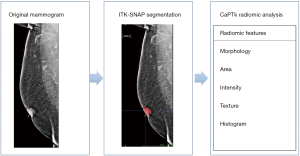
The evaluation of the axilla by mammography can be limited due to the body habitus of most men, lack of the ability to identify the SLN amongst the number of potentially visualized nodes by mammagraphy and lack of specific guidelines to include the entire axilla during mammographic examinations. Therefore, we were unable to directly address this question from the reviewer. This topic may be better addressed in the future using MRI or US where the entire axilla could be visualized.
Radiomics analysis
CaPTk (22) is an open source software platform, (https://www.med.upenn.edu/cbica/captk/) that was used for feature extraction of ROIs obtained following ITK-SNAP extraction of tumor lesions. These segmented ROIs were then transferred to CaPTk for radiomics analysis (Table 1). While CaPTk can perform both 2D and 3D radiomic analysis, we performed only 2D radiomic analysis. The radiomic metrics quantifies the characteristics of the region of the interest such as the spatial distribution of the grey-levels making the ROI (shape/size) and the complex relationship between pixels/voxels making up the ROI (texture) using sophisticated data characterization algorithms. Some of the key metrics featured within the CaPTk radiomics platform include first-order statistical metrics of texture such as intensity, histogram; first-order statistical metrics of morphology such as shape, size; second-order statistical metrics of texture such as grey level co-occurrence matrix (GLCM), grey level size zone matrix (GLSZM), grey level run length matrix (GLRLM); and higher-order statistical metrics of texture such as local binary patterns (LBP), Lattice-transformation etc. First-order statistical metrics quantify only the intensity of the signal within a ROI. First order statistical features describe the distribution of individual voxel values without concern for spatial relationships. These are histogram-based properties reporting the mean, median, maximum, minimum values of the voxel intensities on the image, as well as their skewness (asymmetry), kurtosis (flatness), uniformity, and randomness (entropy).
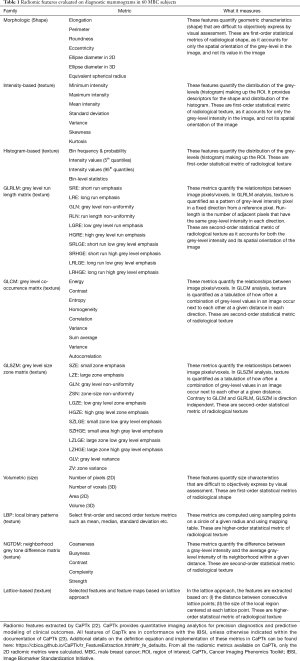
Full table
These radiomic features assess different imaging characteristics. They may be representative of or be surrogates for specific biologic processes but these would also be dependent on clinical and patient specific factors. Radiomics therefore may potentially enhance or nuance the clinical and pathological data available for treating physicians to make patient specific decisions.
Second-order statistical metrics take into consideration the intensity as well its spatial orientation, location within a ROI. Higher-order metrics transform the image to provide additional information regarding frequency, assessment at multiple levels (local versus global assessment). Post-radiomics analysis, all results were exported in comma separated value (.csv) format for radiomic analysis and correlation with pathological node positive and node negative status in all 60 subjects with a confirmed diagnosis of invasive breast carcinoma.
Statistical analysis
An independent t-test or Wilcoxon rank sum test were used, depending on data normality, to compare radiomic metrics that would produce statistically notable differences between pN0 (node negative) and pNmic (node with a micromet defined as tumor <2 mm)/N1a (tumor spread to 1–3 lymph nodes) using MLO and CC mammograms, separately. Graphical approach was used to illustrate the pattern of radiomic signals with differences between clinical findings. Since the main purpose of this study is to discover signal patterns by radiomics categories, we have used unadjusted P value to filter features for graphical illustration. Percent of features with unadjusted P<0.05 under each radiomic categories were calculated. ElasticNet was used to further explore important predictors and the overall prediction accuracy. Leave-one-out (LOO) procedure was used to validate performance. The predicted probability from LOO was used to calculate AUC. We have counted number of times a feature been selected by ElasticNet as the important predictor from each iteration of LOO, and then rank all features by this count. SAS9.4 (SAS, NC, USA) was used for statistical analysis.
Results
A total of 60 patients met the inclusion criteria. The cohort characteristics are found in Tables 2,3. The final cohort included 28/60 (46%) patients who were pathologically node positive and 32/60 (53%) patients who were pathologically node negative (Table 1). Among the tumors, 41/60 (68%) were low-grade (Bloom Richardson Score grades 1–2) and 17/60 (28%) were high-grade (Bloom Richardson Score grade 3). Based on histopathology by IHC, 30/60 (50%) were luminal A and 25/60 (42%) were luminal type B. Five patients had indeterminate histopathology. Mean age was 66.2 years (range, 40–91) years. Mean tumor size was 1.9 (range, 0.7–4.5) cm. Node positive patients include patients who were pathologically N1mic, N1, N2, N3. Our cohort included 5 patients with N1mic, 16 patients with N1, 3 patients with N2 disease, and 4 patients with N3.
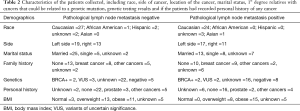
Full table
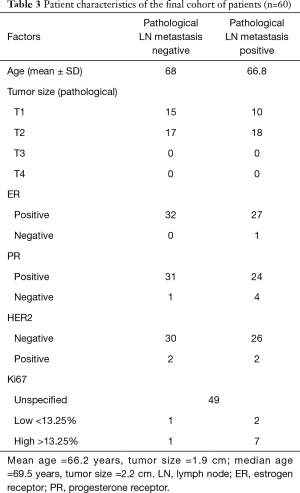
Full table
The ElasticNet based receiver operating characteristic (ROC) curves showed an AUC of 0.52; 95% CI (0.37–0.68) and 0.71 95% CI (0.57–0.85) in the testing cohort to differentiate patients with pathological node negative vs. pathologically node positive disease based upon analysis of the primary tumor lesion (Figure 3).
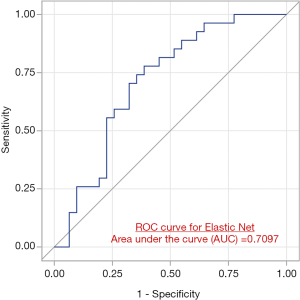
Radiomic results
The tumor characteristics using radiomic metrics available in CaPTk software of highest significance is shown in Table 4. These metrics were derived from the MLO and CC mammogram of each patient. For clarity purposes, we only reported those variables that were statistically significant (P<0.05). The violin plots in Figures 4,5 show different distributions in radiomic properties between pN0 and pN1, respectively, and these distributions are similarly illustrated with boxplots in Figures S1,S2.
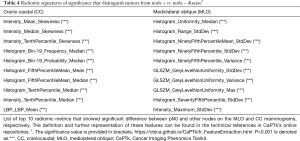
Full table
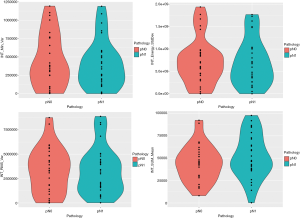
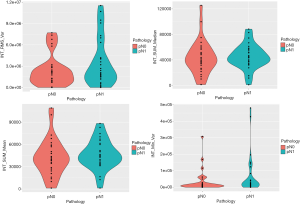
Interestingly, all of them belonged to first-order texture metrics.
A total of 276 out of 1,360 radiomic metrics were found to be significantly (unadjusted P<0.05) different between the two groups. The best performing metrics were mostly (80%) based on first-order texture approaches i.e., histogram and intensity (Table 4).
Discussion
The incidence of MBC has been rising over the past few decades (24). There is an increasing appreciation of differences in the tumor biology of FBC versus MBC (25), thereby highlighting the need for studies focused on this unique population. Currently, there is a lack of studies supporting the treatment recommendations for MBC. What is clear is that after correcting for confounding features, MBC patients have a worse prognosis, stage for stage (1). Therefore, it is imperative to focus on this subset of breast cancer patients and consider tailored strategies such as neoadjuvant therapy or omitting sentinel nodes evaluation, if feasible. ALN status is an important factor in developing a personalized treatment plan for female patients with breast cancer (7), and since 40% of MBCs have pathological detected nodal involvement (1), it would optimize management to reliably know nodal status at the time of diagnosis.
Non-invasive prediction of ALN metastasis preoperatively has significant clinical impact. Currently, ultrasound has a specificity for positivity of 75–86% in all patients and is non-invasive. However, an US study identifying a suspicious node would prompt a US guided biopsy which requires additional time delay due to the need for another appointment and a false negative rate up to 30% (16).
Surgery is still needed to definitively confirm the status of the ALN if preoperative nodal status is not established. These are invasive procedures and associated with sequelae such as seroma, arm pain, infection and lymphedema (26,27). Sonographically guided biopsy of nodes has variable reliability (28). Since mammography is already used as a diagnostic tool and current workflow would be enhanced, we chose mammography for our study.
This study explored the radiomic differences between tumors in pN0 vs. non-pN0 subjects. We identified 276 out of 1,360 radiomic metrics to be significantly (P<0.05) between these two groups of patients. These radiomic features indicates that the distribution of grey levels within a ROI (indicative of radiological texture) is different in primary tumors that are node negative versus those that are node positive. We have reported the predictive value of radiomics in terms of the AUC metric in Figure 3. We report an AUC of 0.71 in discriminating pathologically node negative vs. node positive male patients using radiomic-metrics derived from mammograms. Genomic information was not available for this cohort of patients therefore not evaluated in this study.
Of the 276 radiomic metrics that were found to be significantly (P<0.05) different between the pN0 and non-pN0 subjects, the best performing metrics were based on first-order texture histogram and intensity. The observation of first-order metrics to be significantly different between the two groups i.e., pN0 vs. non-pN0 nodes is encouraging, as first order metrics are easy to implement in the existing clinical workflow without any additional computational or technical complications. A number of clinical imaging software by default provides histograms or ROIs from where these metrics can be easily derived.
To improve the generalization ability and optimize the model, we used the ElasticNet method in conjunction with LOO cross-validation to build a prediction model. Our findings indicated that our prediction model has a moderate discrimination power (AUC =0.71) to distinguish between patients with pathological node negative vs. pathologically node disease based on radiomic analysis of the primary tumor lesion using their MLO view, the performance of the prediction mode using the CC view was comparatively lower (AUC =0.52). Interestingly, all the CC and MLO-based radiomic metrics that showed significant difference between pN0 and other nodes on the MLO and CC mammograms, respectively (Table 4), also were highly ranked as important variables that dictated the performance of the ElasticNet -based prediction model. This observation increases our confidence in the reliability of important predictors to discriminate patients with pathological node negative vs. pathologically node disease based on radiomic analysis of the primary tumor lesion.
There were different radiomic features in the tumors between the CC and MLO views which can be explained by the fact that imaging features can be different in CC and MLO views influencing the textural features that are significant. In their study (29), exploring mammographic structural features (texture/parenchymal pattern) jointly for the improved risk segregation of screening women, Winkel et al., reported that a more precise density measures were achieved when mentally fusing two projections (CC and MLO) compared with assessing only a single projection of the breast, which aided in improved risk assessment. Therefore, it is possible that the significant texture metrics assessed from the two different views may be different, due to the variation in the breast tissue orientation in the different views. Further, creating a separate model using CC and MLO data separately had the increased impact of our study, as researchers with only CC or MLO view could still benefit from our results.
Zheng et al. demonstrated that deep learning radiomics could yield a 90% accuracy of predicting metastatic ALN in early stage FBC using ultrasound and shear wave elastography via deep learning approaches (8). Using a radiomics signature of 10 radiomic metrics, one non-wavelet feature and nine wavelet features, which were significantly related to lymph node metastasis, Yang et al., showed the feasibility of using mammogram-derived texture metrics in preoperatively predicting lymph node status (30). It is encouraging to see the similar observations in MBC in our current study as well.
For MBC, standard treatment has been mastectomy with axillary node dissection for clinically node positive patients or sentinel node biopsy with selective axillary dissection based on the results of the sentinel node biopsy (1). There is no data on the role of neoadjuvant chemotherapy in MBC patients due to the small number of cases in individual centers. Hypothetically, accurate nodal status could allow consideration of downstaging the axilla in clinically node positive patients leading to limited axillary surgery with less morbidity if the negative predictive value was high. Neoadjuvant endocrine therapy and its role is unknown in males; however, accurate nodal information preoperatively could increase the opportunity for such a clinical trial. Further validation would be needed in patients who had negative axilla by radiomics if sentinel node biopsy could be omitted. As 50% of MBC patients are node negative, only 18–25% of MBC patients undergo SLN surgery alone or prior to axillary node dissection. Therefore, having an adjunct tool to predict nodal involvement that is non-invasive which can establish nodal status would be desirable.
There are limitations to our study as it is based on retrospective data. The mammography images are from three different centers however their acquisition protocol comparable. The distribution of images is 43% from facility 1, 33% from facility 2 and finally 23% from facility 3. Again, with MBC being such a rare disease, pooling data becomes essential. Some differences may be attributed to differences in imaging technique. Additionally, segmenting lesions manually can be another source of subjectivity that can affect the analysis. Since this study is a retrospective study, there was some demographic information that was not procured. Finally, due to the rarity of MBC, the number of cases are small compared to a study that would assess FBC.
Conclusions
Our pilot study suggests that a radiomics-based texture signature of the primary tumor is different between pathologically node negative vs. node positive male patients. Following validation radiomic features of the primary tumor could be a surrogate for nodal status. Our data merits further investigation in a larger sample size to confirm which patients could forego sentinel node biopsy and identifying additional biological-radiomic correlations to predict early on which MBC patients might be good candidates for neoadjuvant therapy.
Acknowledgments
Funding: None.
Footnote
Reporting Checklist: The authors have completed the MDAR reporting checklist. Available at http://dx.doi.org/10.21037/abs-20-103
Data Sharing Statement: Available at http://dx.doi.org/10.21037/abs-20-103
Peer Review File: Available at http://dx.doi.org/10.21037/abs-20-103
Conflicts of Interest: All authors have completed the ICMJE uniform disclosure form (available at http://dx.doi.org/10.21037/abs-20-103). RK has a patent on breast cancer analysis, and is the CMO and Founder Imaging Endpoints Core Lab, the MIO for ImaginAB, and a consultant for Merrimack, Globavir, Verve, Dracon, TD2, and TGEN/City of Hope. VD is an Advisory Board Member for Deeptek and is a consultant for Radmetrix. The other authors have no conflicts of interest to declare.
Ethical Statement: The authors are accountable for all aspects of the work in ensuring that questions related to the accuracy or integrity of any part of the work are appropriately investigated and resolved. The study was conducted in accordance with the Declaration of Helsinki (as revised in 2013). The study was approved by the Western Institutional Review Board to exempt the ethics review with the exemption number (B4-Exemption-Moorthy). Informed consent was also waived.
Open Access Statement: This is an Open Access article distributed in accordance with the Creative Commons Attribution-NonCommercial-NoDerivs 4.0 International License (CC BY-NC-ND 4.0), which permits the non-commercial replication and distribution of the article with the strict proviso that no changes or edits are made and the original work is properly cited (including links to both the formal publication through the relevant DOI and the license). See: https://creativecommons.org/licenses/by-nc-nd/4.0/.
References
- Leon-Ferre RA, Giridhar KV, Hieken TJ, et al. A contemporary review of male breast cancer: current evidence and unanswered questions. Cancer Metastasis Rev 2018;37:599-614. [Crossref] [PubMed]
- Cardoso F, Bartlett JMS, Slaets L, et al. Characterization of male breast cancer: results of the EORTC 10085/TBCRC/BIG/NABCG International Male Breast Cancer Program. Ann Oncol 2018;29:405-17. [Crossref] [PubMed]
- Ahmed M, Purushotham AD, Douek M. Novel techniques for sentinel lymph node biopsy in breast cancer: a systematic review. Lancet Oncol 2014;15:e351-62. [Crossref] [PubMed]
- Lucci A, McCall LM, Beitsch PD, et al. Surgical complications associated with sentinel lymph node dissection (SLND) plus axillary lymph node dissection compared with SLND alone in the American College of Surgeons Oncology Group Trial Z0011. J Clin Oncol 2007;25:3657-63. [Crossref] [PubMed]
- Alvarez S, Añorbe E, Alcorta P, et al. Role of sonography in the diagnosis of axillary lymph node metastases in breast cancer: a systematic review. AJR Am J Roentgenol 2006;186:1342-8. [Crossref] [PubMed]
- Wu S, Zheng J, Li Y, et al. A radiomics nomogram for the preoperative prediction of lymph node metastasis in bladder cancer. Clin Cancer Res 2017;23:6904-11. [Crossref] [PubMed]
- Ouldamer L, Arbion F, Balagny A, et al. Validation of a breast cancer nomogram for predicting nonsentinel node metastases after minimal sentinel node involvement: Validation of the Helsinki breast nomogram. Breast 2013;22:787-92. [Crossref] [PubMed]
- Zheng X, Yao Z, Huang Y, et al. Deep learning radiomics can predict axillary lymph node status in early-stage breast cancer. Nat Commun 2020;11:1236. [Crossref] [PubMed]
- Gierach GL, Li H, Loud JT, et al. Relationships between computer-extracted mammographic texture pattern features and BRCA1/2 mutation status: a cross-sectional study. Breast Cancer Res 2014;16:424. [PubMed]
- Tamez-Peña JG, Rodriguez-Rojas JA, Gomez-Rueda H, et al. Radiogenomics analysis identifies correlations of digital mammography with clinical molecular signatures in breast cancer. PLoS One 2018;13:e0193871 [Crossref] [PubMed]
- Saha A, Harowicz MR, Grimm LJ, et al. A machine learning approach to radiogenomics of breast cancer: a study of 922 subjects and 529 DCE-MRI features. Br J Cancer 2018;119:508-16. [Crossref] [PubMed]
- Tan H, Wu Y, Bao F, et al. Mammography-based radiomics nomogram: a potential biomarker to predict axillary lymph node metastasis in breast cancer. Br J Radiol 2020;93:20191019 [Crossref] [PubMed]
- Dong Y, Feng Q, Yang W, et al. Preoperative prediction of sentinel lymph node metastasis in breast cancer based on radiomics of T2-weighted fat-suppression and diffusion-weighted MRI. Eur Radiol 2018;28:582-91. [Crossref] [PubMed]
- Guo X, Liu Z, Sun C, et al. Deep learning radiomics of ultrasonography: Identifying the risk of axillary non-sentinel lymph node involvement in primary breast cancer. EBioMedicine 2020;60:103018 [Crossref] [PubMed]
- Kontos D, Winham SJ, Oustimov A, et al. Radiomic phenotypes of mammographic parenchymal complexity: toward augmenting breast density in breast cancer risk assessment. Radiology 2019;290:41-9. [Crossref] [PubMed]
- Solon JG, Power C, Al-Azawi D, et al. Ultrasound-guided core biopsy: an effective method of detecting axillary nodal metastases. J Am Coll Surg 2012;214:12-7. [Crossref] [PubMed]
- Meng L, Dong D, Chen X, et al. 2D and 3D CT radiomic features performance comparison in characterization of gastric cancer: a multi-center study. IEEE J Biomed Health Inform 2020; Epub ahead of print. [Crossref] [PubMed]
- Shen C, Liu Z, Guan M, et al. 2D and 3D CT radiomics features prognostic performance comparison in non-small cell lung cancer. Transl Oncol 2017;10:886-94. [Crossref] [PubMed]
- Yushkevich PA, Piven J, Hazlett HC, et al. User-guided 3D active contour segmentation of anatomical structures: significantly improved efficiency and reliability. NeuroImage 2006;31:1116-28. [Crossref] [PubMed]
- Zhang HX, Sun ZQ, Cheng YG, et al. A pilot study of radiomics technology based on X-ray mammography in patients with triple-negative breast cancer. J Xray Sci Technol 2019;27:485-92. [Crossref] [PubMed]
- Huang YQ, Liang CH, He L, et al. Development and validation of a radiomics nomogram for preoperative prediction of lymph node metastasis in colorectal cancer. J Clin Oncol 2016;34:2157-64. [Crossref] [PubMed]
- Davatzikos C, Rathore S, Bakas S, et al. Cancer imaging phenomics toolkit: quantitative imaging analytics for precision diagnostics and predictive modeling of clinical outcome. J Med Imaging (Bellingham) 2018;5:011018 [Crossref] [PubMed]
- Zwanenburg A, Vallières M, Abdalah MA, et al. The image biomarker standardization initiative: standardized quantitative radiomics for high-throughput image-based phenotyping. Radiology 2020;295:328-38. [Crossref] [PubMed]
- Speirs V, Shaaban AM. The rising incidence of male breast cancer. Breast Cancer Res Treat 2009;115:429-30. [Crossref] [PubMed]
- Piscuoglio S, Ng CKY, Murray MP, et al. The genomic landscape of male breast cancers. Clin Cancer Res 2016;22:4045-56. [Crossref] [PubMed]
- Kootstra J, Hoekstra-Weebers JEHM, Rietman H, et al. Quality of life after sentinel lymph node biopsy or axillary lymph node dissection in stage I/II breast cancer patients: a prospective longitudinal study. Ann Surg Oncol 2008;15:2533-41. [Crossref] [PubMed]
- Fleissig A, Fallowfield LJ, Langridge CI, et al. Post-operative arm morbidity and quality of life. Results of the ALMANAC randomised trial comparing sentinel node biopsy with standard axillary treatment in the management of patients with early breast cancer. Breast Cancer Res Treat 2006;95:279-93. [Crossref] [PubMed]
- Cools-Lartigue J, Sinclair A, Trabulsi N, et al. Preoperative axillary ultrasound and fine-needle aspiration biopsy in the diagnosis of axillary metastases in patients with breast cancer: predictors of accuracy and future implications. Ann Surg Oncol 2013;20:819-27. [Crossref] [PubMed]
- Winkel RR, von Euler-Chelpin M, Nielsen M, et al. Mammographic density and structural features can individually and jointly contribute to breast cancer risk assessment in mammography screening: a case-control study. BMC Cancer 2016;16:414. [Crossref] [PubMed]
- Yang J, Wang T, Yang L, et al. Preoperative prediction of axillary lymph node metastasis in breast cancer using mammography-based radiomics method. Sci Rep 2019;9:4429. [Crossref] [PubMed]
Cite this article as: Moorthy A, Varghese B, Rajack S, Korn R, Cen S, Moorthy B, Duddalwar V. Predicting nodal status in male breast cancer using mammogram based radiomic metrics. Ann Breast Surg 2021;5:2.